Understanding the Importance of Data Annotation in Business
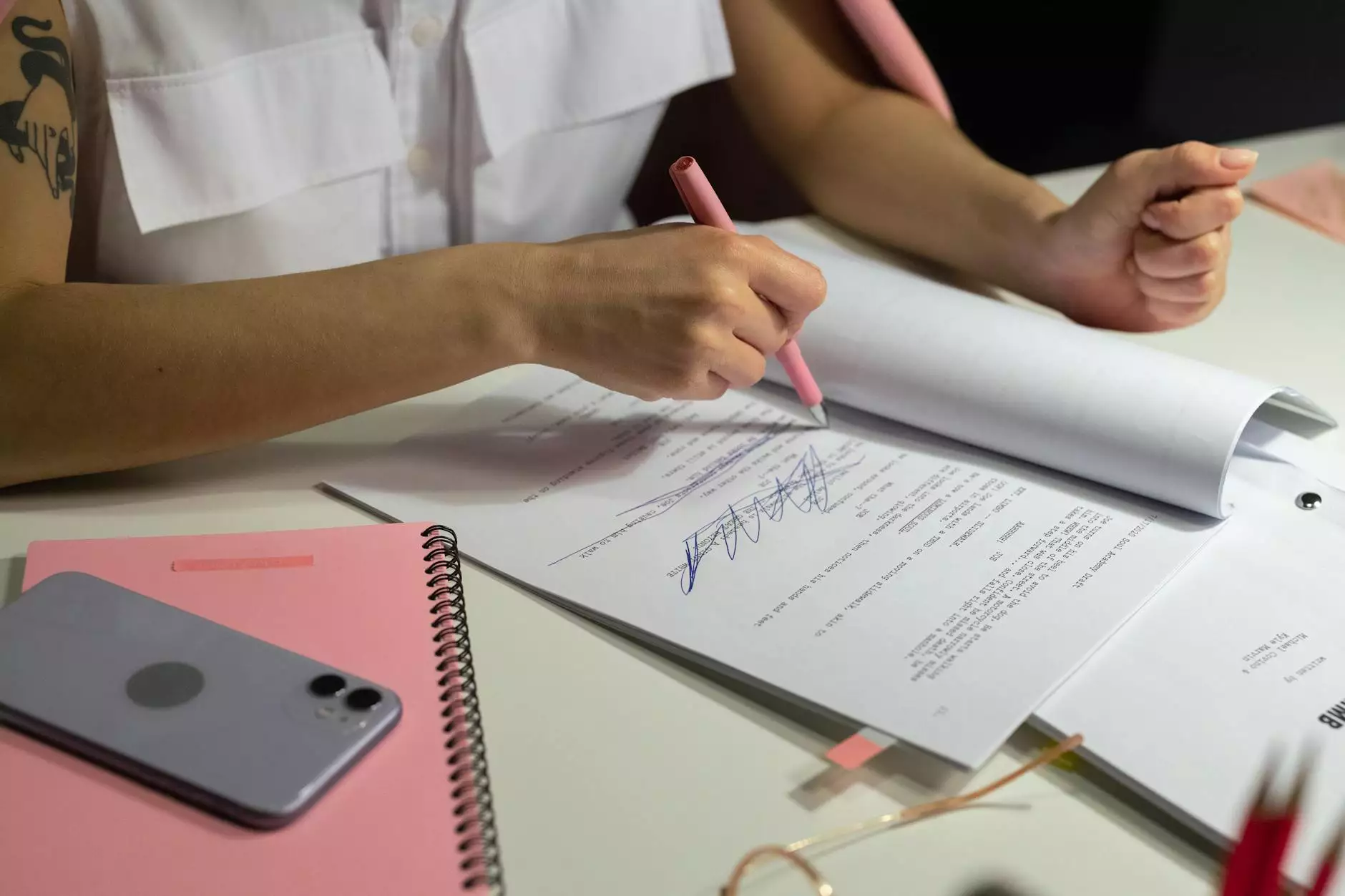
In the rapidly evolving landscape of artificial intelligence and machine learning, the significance of data annotation cannot be overstated. For businesses, especially those leveraging technologies like computer vision, accurate data annotation is critical to achieving high-quality outcomes. This article delves into the various aspects of data annotation, focusing specifically on tools and platforms that utilize bounding boxes, a popular method for annotating images.
What is Data Annotation?
Data annotation is the process of labeling data to train machine learning models. It involves various types of data, including text, images, audio, and video. For computer vision applications, data annotation typically focuses on images and involves categorizing and labeling objects within those images.
Why is Data Annotation Crucial for Businesses?
Businesses today rely heavily on data-driven decisions. The quality and precision of this data directly affect their operational efficiency and strategic initiatives. Annotated data enables machines to understand input patterns and make informed predictions. Here are some reasons why data annotation is crucial:
- Enhances Model Accuracy: Proper data annotation improves the accuracy of machine learning models, leading to better performance in real-world applications.
- Improves Automation: With well-annotated data, businesses can automate processes efficiently, saving time and reducing human error.
- Facilitates AI Training: High-quality annotated data is essential for training AI systems, helping them learn from patterns and make decisions.
- Supports Diverse Applications: Data annotation supports various applications, from autonomous vehicles to healthcare diagnostics and retail analytics.
Types of Data Annotation Techniques
There are several data annotation techniques available, each serving specific purposes based on the type of data and the machine learning model being developed. Let’s explore some common techniques:
1. Bounding Boxes
Bounding boxes are a widely used method for object detection in images. This technique involves drawing a rectangular box around an object in an image to indicate its location. Bounding boxes help machine learning algorithms recognize objects in a two-dimensional space, making them invaluable for applications such as:
- Self-driving cars
- Facial recognition
- Image classification
2. Polygon Annotation
This method is more precise than bounding boxes, as it allows the annotation of irregularly shaped objects. You can outline the object with multiple points, providing much-needed detail.
3. Semantic Segmentation
This technique involves dividing an image into regions with the same attributes. Each pixel is labeled, providing fine-grained details that help in recognizing objects at a pixel level.
The Role of Data Annotation Tools and Platforms
As the demand for annotated data grows, so does the need for sophisticated data annotation tools and platforms. These tools ease the annotation process, making it faster and more accurate.
Key Features of Top Data Annotation Tools:
- User-Friendly Interface: A simple interface allows annotators to work efficiently without a steep learning curve.
- Collaboration Features: Many platforms offer collaborative features where multiple users can annotate data simultaneously, enhancing productivity.
- Quality Control Mechanisms: Quality assurance is crucial. Top tools incorporate robust validation processes to maintain high annotation standards.
- Integration Capabilities: The ability to integrate with existing workflows and machine learning pipelines is essential for seamless operations.
Top Data Annotation Tools and Platforms
When it comes to selecting the right data annotation tool or platform, several options stand out in the market. Here are some of the top contenders known for their efficiency and reliability:
Keylabs.ai - A Premier Data Annotation Platform
Keylabs.ai is a leading platform that specializes in providing data annotation tools designed to cater to modern business needs. They utilize advanced algorithms and a team of skilled annotators to ensure the highest quality of annotated data. The following features make Keylabs.ai stand out:
- Automated and Manual Annotation: Keylabs.ai offers a hybrid model where businesses can choose between automated and manual data annotation, ensuring flexibility according to their requirements.
- Real-time Collaboration: Teams can work together seamlessly on projects, accelerating the annotation process.
- Customizable Workflows: Users can design workflows tailored to their specific needs, aligning with their existing project management systems.
Labelbox
Labelbox is another robust data annotation tool that focuses on simplifying the annotation process while maintaining a high quality. It offers features such as:
- Project Management Tools: Helps teams manage multiple annotation projects efficiently.
- AI-Assisted Annotation: Uses machine learning to suggest annotations, speeding up the process.
SuperAnnotate
SuperAnnotate is renowned for its emphasis on quality control. Some of its key features include:
- Custom Quality Assurance: Allows businesses to set their quality benchmarks.
- Multi-format Support: Supports various types of data, including images, videos, and text.
Best Practices for Data Annotation
To maximize the benefits gained from data annotation, businesses should adhere to certain best practices:
1. Define Clear Annotation Guidelines
Guidelines should be easy to understand and provide a clear direction for annotators. This reduces ambiguity and maintains consistency across the dataset.
2. Regularly Train Annotators
Continuous training ensures that annotators are well-versed in the latest techniques and tools, which enhances the quality of the annotated data.
3. Use Quality Checks
Incorporate multiple rounds of quality checks to ensure the validity of the annotations. This can prevent costly errors in the training data.
4. Stay Updated with Technological Advances
As technologies evolve, so should your annotation tools and methods. Regularly evaluating and updating your processes can lead to substantial improvements.
The Future of Data Annotation
As AI technology advances, so too will the methods and tools used for data annotation. Here are some trends to watch in the data annotation landscape:
- Increased Automation: With the advancements in AI, automated systems for annotation will become more robust, requiring less human intervention.
- Focus on Quality over Quantity: Businesses will shift towards obtaining high-quality annotated data rather than simply amassing large datasets.
- Integration with Machine Learning Pipelines: Annotation tools will increasingly integrate with machine learning workflows, allowing for a seamless transition from data annotation to model training.
Conclusion
In conclusion, data annotation plays an invaluable role in the success of machine learning and AI applications. By utilizing powerful tools and adhering to best practices, businesses can significantly enhance the quality of their data. The importance of techniques like bounding boxes cannot be underscored enough, as they are fundamental in various computer vision applications. Tools like Keylabs.ai are leading the charge in providing efficient solutions that cater to these needs.
As the field of data annotation continues to evolve, understanding its nuances will position businesses to leverage AI capabilities to their fullest potential. Investing in reliable data annotation is no longer an option; it is essential for remaining competitive in an increasingly data-driven world.