Unlocking the Power of Agriculture Datasets for Machine Learning
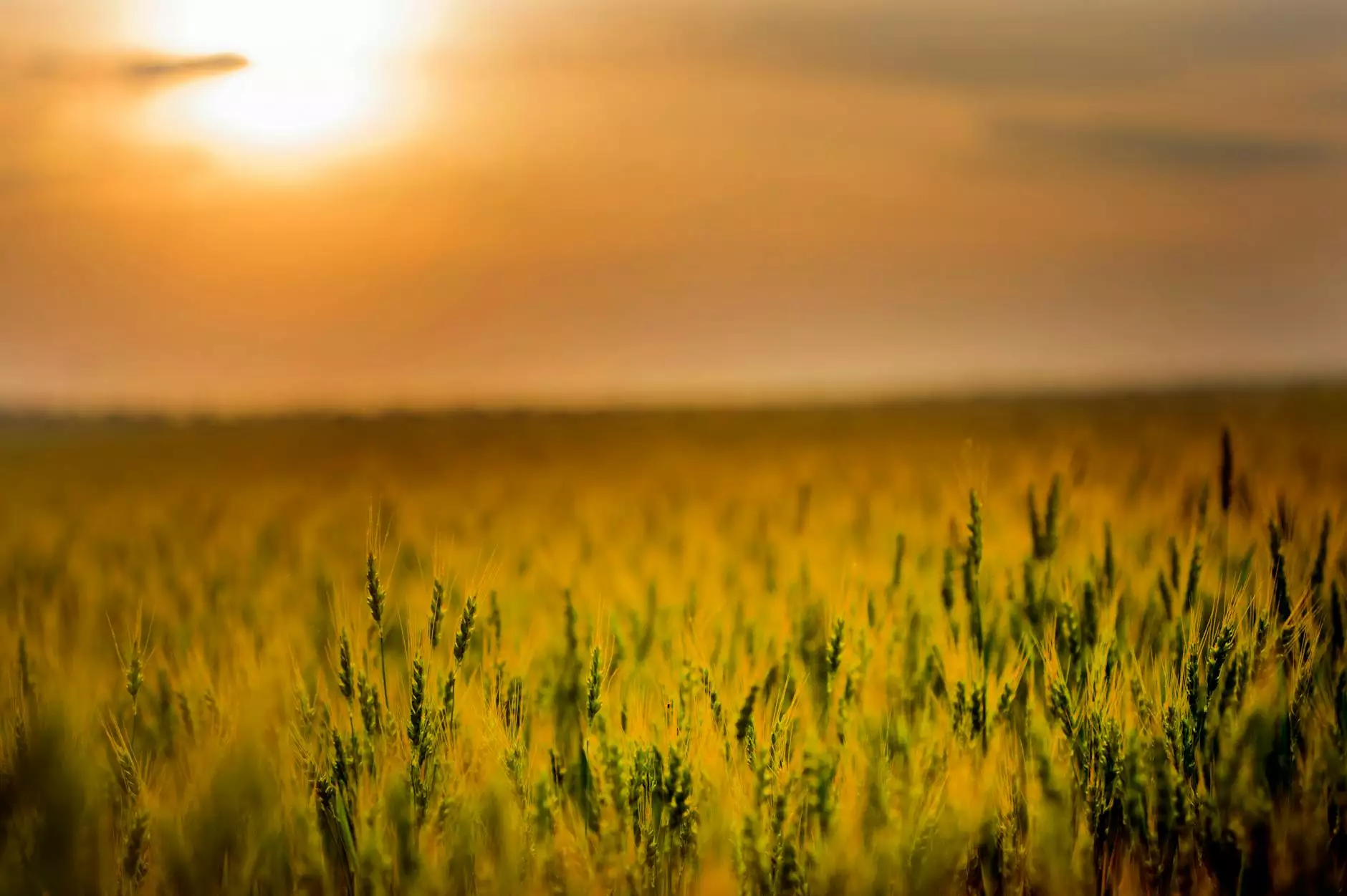
The agricultural industry is at a pivotal moment, where tradition meets technology. As we navigate through the complexities of food production and sustainability, the role of machine learning becomes increasingly vital. One of the most essential components driving this innovation is the availability and utilization of agriculture datasets for machine learning.
The Importance of Agriculture Datasets
In the context of machine learning, agriculture datasets serve multiple roles. They are the backbone of predictive analytics, enabling farmers and agricultural scientists to make decisions based on empirical data rather than intuition alone. These datasets encompass a variety of elements, including climate data, crop yields, soil types, pest occurrences, and more.
Types of Agriculture Datasets
Exploring the types of datasets available is essential for understanding how they can be leveraged for machine learning:
- Soil Data: Includes properties such as pH, moisture content, and nutrient levels.
- Weather Data: Historical and predictive insights into climatic conditions impacting crops.
- Crop Yield Data: Tracks performance metrics of various crops over time.
- Pest and Disease Data: Information on pest populations and disease outbreaks for different crops.
- Satellite Imagery: Used for monitoring large agricultural areas and assessing crop health.
How Machine Learning Transforms Agriculture
The integration of machine learning with agriculture datasets is revolutionizing how farms operate. With the help of advanced algorithms, farmers can gain insights that were previously unattainable. Here are some of the transformative impacts:
1. Precision Agriculture
Precision agriculture leverages data analytics to optimize field-level management regarding crop farming. By utilizing agriculture datasets for machine learning, farmers can:
- Enhance resource efficiency by applying water, nutrients, and pesticides only where needed.
- Increase yield by understanding the best conditions for crop production.
- Reduce costs and improve profitability through better planning and process efficiencies.
2. Predictive Analytics for Yield Forecasting
By analyzing historical data, machine learning models can predict future crop yields with remarkable accuracy. This capability allows:
- Farmers to plan better for harvesting and storage.
- Supply chain stakeholders to optimize logistics and distribution.
- Investors to make informed decisions regarding funding and financial backing.
Building Machine Learning Models with Agriculture Datasets
Data Collection and Preparation
The first step in building an effective machine learning model is the collection and preparation of data. This involves:
- Gathering raw data from various sources such as sensors, weather stations, and satellite observations.
- Cleaning the data by removing inconsistencies, duplicates, and irrelevant information.
- Transforming the data into a format that can be utilized by machine learning algorithms.
Model Selection and Training
Once the data is ready, the next stage involves selecting an appropriate model based on the kind of predictions or classifications needed. Popular models in agricultural applications include:
- Decision Trees: Great for classification tasks based on various input features.
- Support Vector Machines: Useful for complex classification tasks.
- Neural Networks: Effective for pattern recognition, especially in large datasets.
After selecting the model, training it on the dataset entails feeding the model examples so it can learn to make accurate predictions.
Model Evaluation
With a trained model, it is crucial to evaluate its performance using metrics such as:
- Accuracy: How often the model makes correct predictions.
- Precision and Recall: Measures the model's ability to identify relevant instances.
- F1 Score: A balance between precision and recall, indicating robustness.
Real-world Applications of Agriculture Datasets in Machine Learning
The potential applications of agriculture datasets for machine learning are vast and varied. Here are a few noteworthy examples:
Crop Disease Detection
Machine learning can be employed to analyze images of plants to detect early signs of disease. By training models on large datasets of healthy and diseased plants, farmers can receive timely alerts, leading to prompt action.
Soil Health Monitoring
Using sensor data, machine learning models can continuously assess soil health parameters. This monitoring allows farmers to create targeted interventions, thus optimizing soil management practices.
Harvest Timing Optimization
Machine learning algorithms can forecast the optimal harvest time by analyzing environmental conditions over a period. This insight helps farmers maximize yield quality and quantities, ensuring better product offerings in the market.
The Future of Agriculture and Machine Learning
The future of agriculture appears bright with the integration of machine learning and agriculture datasets. As technology continues to evolve, the following trends are expected:
1. Increased Collaboration Across Disciplines
Collaboration between data scientists, agronomists, and farmers will facilitate the creation of more customized and effective machine learning models adapted to unique farming environments.
2. Enhanced Data Accessibility
With advancements in technology, the availability of agricultural datasets will increase. Open data platforms will empower more farmers to utilize machine learning, providing tools for better decision-making.
3. Sustainable Practices
Machine learning's ability to analyze data for resource efficiency will promote sustainable practices, reducing the ecological footprint of agriculture while also boosting productivity.
Conclusion
In conclusion, the utilization of agriculture datasets for machine learning is not just a trend; it is a vital step towards a more efficient, productive, and sustainable agricultural future. By embracing these technologies, farmers can not only increase their productivity but also contribute positively to environmental preservation and food security worldwide. As we look ahead, it is clear that those who harness the power of data and machine learning will be at the forefront of the agricultural revolution.
agriculture dataset for machine learning