Why a Data Labeling Platform is Crucial for Your Business Success
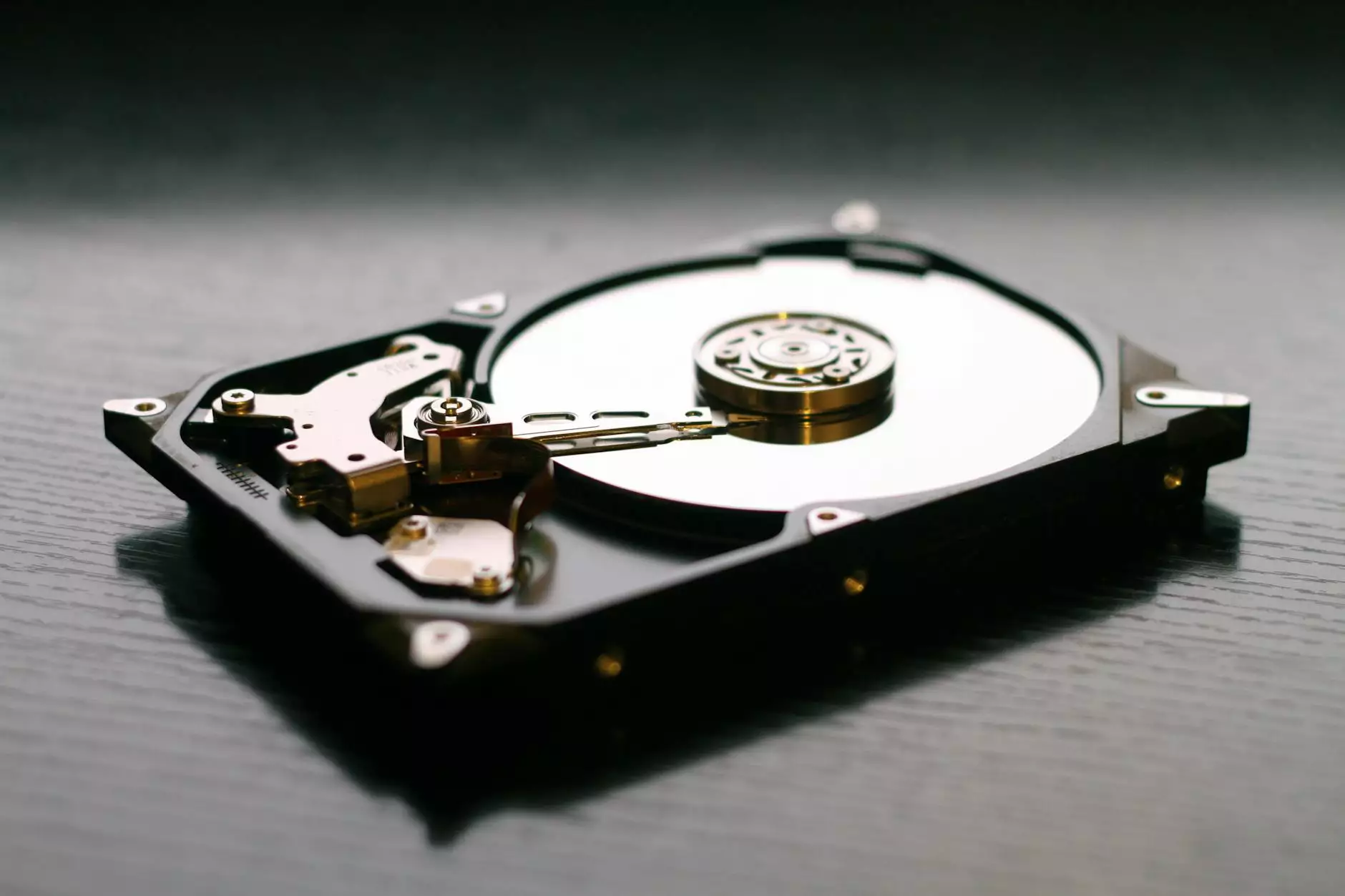
The digital age has ushered in a torrent of data from various sources. This information can be overwhelming, yet it holds the power to drive innovation and efficiency in businesses. However, to harness this potential, companies must focus on the critical task of data labeling. A reliable data labeling platform can be the cornerstone of your machine learning and artificial intelligence initiatives. Understanding the intricacies of data annotation tools will provide businesses with a competitive edge that is hard to ignore.
Understanding Data Labeling
Data labeling refers to the process of tagging data with meaningful labels that allow algorithms to learn from it. Whether it's images, video clips, or text, the classification of data enables businesses to develop models that can make predictions, automate tasks, or even understand customer behavior. But why is this important?
The Significance of Data Annotation
Data annotation is necessary for several reasons:
- Training Machine Learning Models: Algorithms learn from labeled data. Annotated datasets are essential for the training of models that power applications from autonomous vehicles to virtual assistants.
- Improving Accuracy: High-quality data leads to accurate predictions. A well-organized labeling process ensures that machine learning models perform their intended tasks with precision.
- Facilitating Compliance: In sectors like healthcare and finance, precise data labeling is crucial for regulatory compliance and ethical use of AI technologies.
The Role of Data Labeling Platforms
To effectively manage the data labeling process, businesses must invest in a dedicated data labeling platform. Here’s how these platforms are transforming the data annotation landscape:
Streamlining Workflows
A robust data labeling platform offers tools that streamline the data annotation workflow. This includes the ability to manage large volumes of data efficiently, enabling teams to keep up with the pace of project demands. By using a data annotation tool, businesses can significantly reduce the time spent on manual labeling.
Enhancing Collaboration
These platforms foster collaboration among data scientists, annotators, and project managers. Features such as real-time updates and comment sections facilitate communication, ensuring everyone is on the same page. This collaborative approach enhances the quality of the output.
Quality Control Mechanisms
Quality control is paramount in data annotation. Effective platforms provide mechanisms for reviewing labeled data, thus ensuring accuracy. This can include peer reviews, automated checks, and client feedback options. High-quality labeled data is indispensable for the success of any machine learning project.
Key Features of a Data Labeling Platform
To maximize the advantages of a data labeling platform, it is essential to understand its key features:
User-Friendly Interface
The easiest platforms to use have a user-friendly interface that minimizes the learning curve for new users. This is critical for maintaining productivity, especially when onboarding new team members.
Custom Annotation Tools
Data labeling platforms often come equipped with a variety of custom annotation tools tailored to specific types of data, such as:
- Image Segmentation: Perfect for applications in computer vision.
- Text Tagging: Useful for natural language processing.
- Video Annotation: Necessary for dynamic content understanding.
Integration Capabilities
A comprehensive data labeling platform should easily integrate with existing tools and systems within an organization. This facilitates a seamless flow of data between different stages of data processing and model training.
Flexibility and Scalability
The growth trajectory of businesses means they require scalable solutions. A quality data labeling platform accommodates increased data loads, ensuring the tasks are handled efficiently without compromising quality.
Choosing the Right Data Labeling Platform
When selecting the best data labeling platform for your organization, consider the following:
- Define Your Needs: Understand the specific requirements of your projects, such as type of data, required accuracy, and label types.
- Research Options: Look for platforms that have a proven track record in your industry.
- Gather Reviews: Seek testimonials and case studies from other companies that have utilized the platform.
- Test Usability: Take advantage of trial periods to evaluate usability and features.
Case Studies of Successful Data Labeling Platforms
To illustrate the impact of effective data labeling, consider the following examples:
Company A – Enhancing Autonomous Vehicles
Company A implemented a leading data labeling platform which allowed them to accelerate the annotation of image data from their autonomous vehicles. By improving the speed and accuracy of their labeled datasets, they were able to advance their model development rapidly, resulting in a 30% decrease in development time.
Company B – Improving Customer Insights
For Company B, moving to a sophisticated data annotation tool allowed them to refine their customer segmentation strategies. By tagging interactions with specific labels that identified customer moods and needs, they developed personalized marketing campaigns that increased engagement by over 50%.
The Future of Data Labeling Platforms
The future holds exciting possibilities for the evolution of data labeling platforms. With advancements in artificial intelligence and machine learning, several trends are emerging:
Automated Annotation Tools
While human annotation will always be necessary for certain tasks, automated tools are becoming increasingly sophisticated. These technologies can significantly reduce the amount of manual work involved, thus expediting the process.
Increased Use of Crowdsourcing
As businesses increasingly understand the value of diverse perspectives, crowdsourcing is becoming popular in data labeling. This approach can expand the labeling workforce and enhance the accuracy of data representation.
Focus on Ethical AI
As organizations strive to implement ethical AI practices, the need for transparent data labeling processes will grow. Companies must ensure not just accurate labeling, but also that the data usage aligns with ethical standards.
Conclusion
Investing in a data labeling platform is no longer optional for businesses looking to leverage the power of machine learning. With the right tools, organizations can transform raw data into valuable insights, allowing for smarter decision-making and enhanced customer experiences. By choosing a platform that aligns with your business goals, you can harness the full potential of your data and gain a significant competitive advantage in today’s fast-paced digital landscape.
In conclusion, as we move further into the data-driven future, understanding and utilizing a data labeling platform will be vital. Companies like KeyLabs exemplify the potential impact of sophisticated data annotation tools. Commit to embracing these technologies, and you will be well-equipped to thrive in the modern business environment.